As Large Language Models (LLMs) continue to push the boundaries of what's possible in natural language processing, they've also introduced new challenges. One of the most significant issues facing these powerful AI systems is the phenomenon known as "hallucination." In this blog post, we'll dive deep into the world of LLM hallucinations, exploring what they are, how they can be measured, and the innovative approaches being developed to mitigate this fascinating yet problematic aspect of AI behavior.
Summary
- LLM hallucination refers to the generation of false or nonsensical information by AI language models.
- Hallucinations can be measured through human evaluation, automated metrics, and specialized datasets.
- Approaches to reduce hallucination include improved training data, architectural modifications, and advanced decoding strategies.
- Ongoing research focuses on enhancing model reliability and developing robust evaluation frameworks.
- Addressing hallucination is crucial for the responsible deployment of LLMs in real-world applications.
What is LLM Hallucination?
LLM hallucination refers to the phenomenon where large language models generate text that is false, nonsensical, or unrelated to the input prompt or context. This can manifest in various ways, such as:
- Fabricating facts or events that never occurred
- Creating non-existent citations or references
- Generating inconsistent or contradictory information within the same response
- Producing fluent but meaningless text (sometimes referred to as "fluent BS")
Hallucinations occur because LLMs are trained to predict the most likely next word or sequence based on patterns in their training data, rather than having a true understanding of the world or access to up-to-date factual information. This can lead to the model confidently generating plausible-sounding but incorrect information.
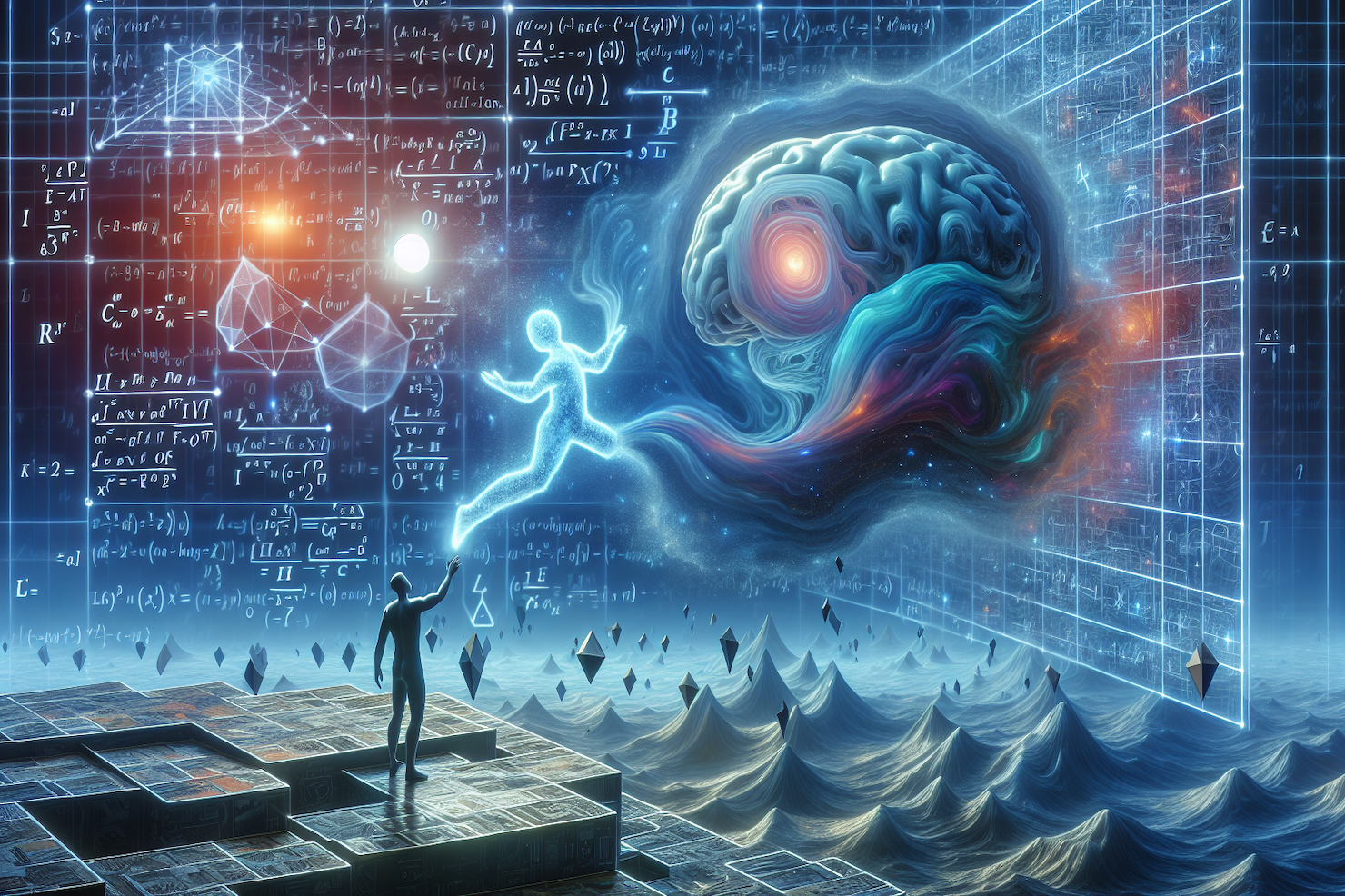
How Can You Measure/Estimate Hallucination?
Measuring and estimating hallucination in LLMs is a complex task that researchers are actively working on. Several approaches have been developed:
- Human Evaluation: Expert reviewers assess model outputs for factual accuracy and coherence. While this method is reliable, it's time-consuming and doesn't scale well.
- Automated Metrics: Researchers have developed metrics like BLEU, ROUGE, and METEOR to evaluate text generation quality. However, these metrics often struggle to capture the nuances of hallucination.
- Fact-Checking Systems: Automated systems that cross-reference model outputs with trusted knowledge bases to identify factual inconsistencies.
- Perplexity and Entropy: These metrics can sometimes indicate when a model is generating improbable or inconsistent text.
- Specialized Datasets: Researchers create datasets with known facts and evaluate how often models generate incorrect information when prompted with questions from these datasets.
- Self-Consistency Checks: Comparing multiple outputs from the same model for a given prompt to identify inconsistencies.
One promising approach is the TruthfulQA benchmark, developed by researchers to specifically evaluate the tendency of language models to generate false or unsupported statements. This benchmark includes a diverse set of questions designed to probe a model's propensity for hallucination.
Approaches to Reduce Hallucination
Researchers and AI developers are exploring various strategies to mitigate hallucination in LLMs:
- Improved Training Data: Curating high-quality, fact-checked datasets for model training to reduce the likelihood of learning and reproducing false information.
- Fact-Aware Training: Incorporating external knowledge bases during the training process to ground the model's outputs in verified facts.
- Architectural Modifications: Developing new model architectures that are less prone to hallucination, such as retrieval-augmented generation models.
- Constrained Decoding: Implementing decoding strategies that guide the model towards more factual and consistent outputs.
- Uncertainty Quantification: Training models to express uncertainty when they are not confident about the information they are generating.
- Multi-Task Learning: Training models on a variety of tasks, including fact-checking and consistency verification, to improve overall reliability.
- Prompt Engineering: Developing prompting techniques that encourage more accurate and factual responses from LLMs.
One particularly interesting approach is the development of "self-aware" language models. These models are trained not only to generate text but also to assess the reliability of their own outputs. For example, researchers at Google AI have experimented with models that can generate text along with confidence scores for each generated token, allowing for more nuanced interpretation of the model's outputs.
The Future of Hallucination Mitigation
As we continue to push the boundaries of LLM capabilities, addressing hallucination remains a critical challenge. Future research directions include:
- Causal Understanding: Developing models with a deeper causal understanding of the world, rather than relying solely on Causal Understanding: Developing models with a deeper causal understanding of the world, rather than relying solely on statistical patterns in text.
- Hybrid AI Systems: Combining neural language models with symbolic AI and knowledge graphs to leverage the strengths of both approaches.
- Continual Learning: Creating models that can update their knowledge base in real-time to maintain accuracy and relevance.
- Explainable AI: Developing techniques to make the reasoning process of LLMs more transparent, allowing for easier identification and correction of hallucinations.
- Ethical Considerations: Addressing the ethical implications of AI-generated misinformation and developing frameworks for responsible AI deployment.
Conclusion
Hallucination in Large Language Models represents both a fascinating insight into the nature of artificial intelligence and a significant challenge for the deployment of these systems in real-world applications. As we continue to explore the vast potential of LLMs, understanding and mitigating hallucination will be crucial for building trustworthy and reliable AI systems.
At A42 Labs, we're at the forefront of research into LLM reliability and safety. Our team is working on innovative approaches to reduce hallucination and improve the overall performance of language models. We believe that by addressing these challenges, we can unlock the full potential of AI to augment human intelligence and drive innovation across industries.
As we move forward, it's clear that the quest to tame the "hallucinating AI" will involve collaboration across disciplines, from machine learning and cognitive science to ethics and philosophy. By continuing to push the boundaries of what's possible while remaining grounded in rigorous scientific inquiry, we can work towards a future where AI systems are not just powerful, but also trustworthy and aligned with human values.
If you're interested in learning more about our work on LLM reliability or how A42 Labs is helping organizations leverage cutting-edge AI technologies responsibly, please don't hesitate to reach out to us at info@a42labs.io.
References
- Lin, S., et al. (2022). TruthfulQA: Measuring How Models Mimic Human Falsehoods. arXiv preprint arXiv:2109.07958. https://arxiv.org/abs/2109.07958
- Xu, J., et al. (2022). How Close is ChatGPT to Human Experts? Comparison Corpus, Evaluation, and Detection. arXiv preprint arXiv:2301.07597. https://arxiv.org/abs/2301.07597
- Evans, O., et al. (2021). Truthful AI: Developing and governing AI that does not lie. arXiv preprint arXiv:2110.06674. https://arxiv.org/abs/2110.06674