In recent years, Generative AI has emerged as a groundbreaking technology, revolutionizing various industries and pushing the boundaries of what artificial intelligence can achieve. This blog post delves into the world of Generative AI, exploring its definition, the innovations driving its rise, different types, use cases, and future prospects.
Summary
- Generative AI refers to AI systems that can create new content, including text, images, music, and more.
- Key innovations driving Generative AI include deep learning, transformer architectures, and large language models.
- Different types of Generative AI include text-to-text, text-to-image, image-to-image, and multimodal models.
- Generative AI has diverse applications across industries, from creative content generation to scientific research and healthcare.
- The future of Generative AI promises more advanced models, ethical considerations, and integration into various aspects of our lives.
What is Generative AI?
Generative AI refers to artificial intelligence systems that can create new content, such as text, images, music, or even video, based on patterns and information learned from existing data. Unlike traditional AI systems that are designed for tasks like classification or prediction, generative AI can produce original outputs that didn't previously exist.
These systems use complex algorithms and neural networks to understand the underlying structure and patterns in their training data, allowing them to generate new content that is similar in style or structure to the original data but unique in its composition.
What innovations have driven the rise of Generative AI?
Several key innovations have contributed to the rapid advancement and widespread adoption of Generative AI:
- Deep Learning: The development of deep neural networks has significantly improved the ability of AI systems to learn complex patterns and representations from large datasets.
- Transformer Architecture: Introduced in 2017, the transformer architecture has revolutionized natural language processing and has been instrumental in creating powerful language models.
- Large Language Models: Models like GPT (Generative Pre-trained Transformer) have demonstrated remarkable capabilities in understanding and generating human-like text.
- Generative Adversarial Networks (GANs): These networks have been crucial in generating realistic images and have applications in various fields, including art and design.
- Transfer Learning: This technique allows models to apply knowledge learned from one task to new, related tasks, significantly reducing the amount of data and training time required.
- Increased Computational Power: Advancements in hardware, particularly GPUs and TPUs, have made it possible to train and run increasingly complex AI models.
Different Types of Generative AI
Generative AI encompasses various types of models, each specialized for different tasks:
- Text-to-Text Models: These models, like GPT-3, can generate human-like text based on prompts or complete partially written text.
- Text-to-Image Models: Systems like DALL-E and Midjourney can create images based on textual descriptions.
- Image-to-Image Models: These models can transform existing images, such as converting sketches to photorealistic images or changing the style of a photograph.
- Music Generation Models: AI systems that can compose original music or create variations on existing compositions.
- Video Generation Models: Emerging models that can create short video clips based on text descriptions or initial frames.
- Multimodal Models: These advanced systems can work with multiple types of data (e.g., text, image, and audio) simultaneously, enabling more complex and varied outputs.
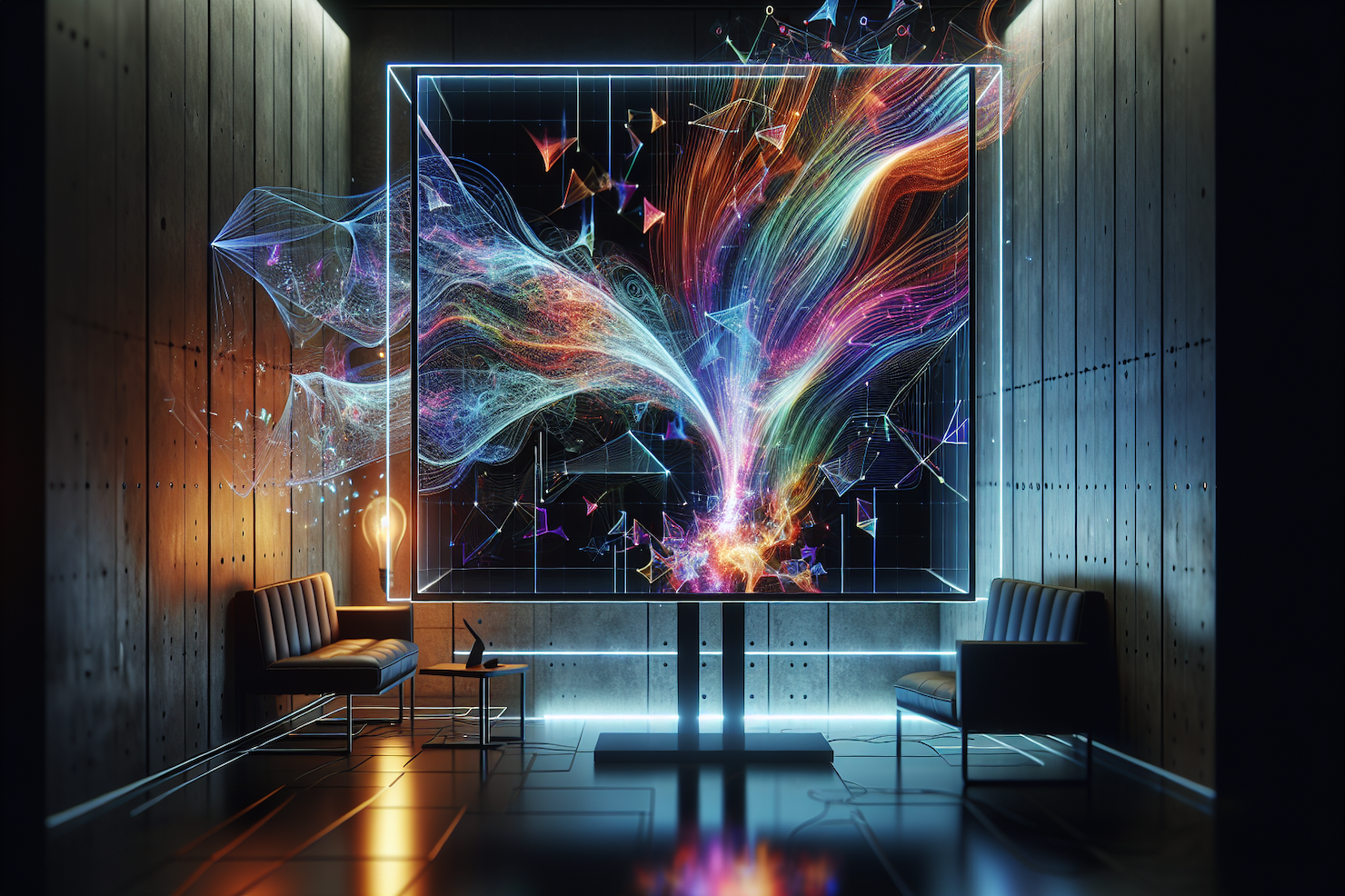
Use Cases of Generative AI
Generative AI has found applications across numerous industries and domains:
- Content Creation: Generating articles, marketing copy, social media posts, and even creative writing.
- Design and Art: Creating unique artwork, graphic designs, and assisting in the creative process for artists and designers.
- Software Development: Assisting programmers by generating code snippets, debugging, and even creating simple programs.
- Healthcare: Generating synthetic medical data for research, assisting in drug discovery, and creating personalized treatment plans.
- Entertainment: Generating scripts, storyboards, and even assisting in music composition and video game design.
- Education: Creating personalized learning materials, generating practice questions, and assisting in curriculum development.
- Customer Service: Powering advanced chatbots and virtual assistants capable of natural, context-aware conversations.
- Scientific Research: Assisting in hypothesis generation, data analysis, and even writing research papers.
Where is Generative AI Headed?
The future of Generative AI is bright and filled with potential:
- More Advanced Models: More Advanced Models: We can expect even more sophisticated models with improved understanding of context, reasoning abilities, and multimodal capabilities.
- Increased Accessibility: As the technology becomes more refined, we'll likely see more user-friendly tools that allow non-experts to harness the power of Generative AI.
- Ethical AI Development: There will be a growing focus on developing ethical guidelines and frameworks to ensure responsible use of Generative AI.
- Integration with Other Technologies: Generative AI will likely be integrated with other emerging technologies like augmented reality, virtual reality, and the Internet of Things.
- Personalization at Scale: Generative AI will enable hyper-personalized experiences across various domains, from education to entertainment.
- Scientific Breakthroughs: The technology could accelerate scientific discoveries by generating hypotheses, designing experiments, and analyzing results.
Conclusion
Generative AI represents a paradigm shift in artificial intelligence, opening up new possibilities across industries and disciplines. As the technology continues to evolve, it promises to revolutionize how we create, innovate, and solve complex problems. However, with great power comes great responsibility, and it's crucial that we approach the development and deployment of Generative AI with careful consideration of its ethical implications and potential societal impacts.
As we stand on the brink of this AI revolution, organizations must stay informed and prepared to leverage Generative AI's potential while navigating its challenges. At A42 Labs, we're committed to helping our clients understand and harness the power of Generative AI in responsible and innovative ways.
In future blogs, we will delve deeper into specific applications of Generative AI and explore best practices for its implementation. If you have any questions or would like to learn more about how A42 Labs is helping organizations with Generative AI, please reach out to us - info@a42labs.io.
References
- Vaswani, A., et al. (2017). Attention Is All You Need. arXiv preprint arXiv:1706.03762. https://arxiv.org/abs/1706.03762
- Brown, T. B., et al. (2020). Language Models are Few-Shot Learners. arXiv preprint arXiv:2005.14165. https://arxiv.org/abs/2005.14165
- Ramesh, A., et al. (2022). Hierarchical Text-Conditional Image Generation with CLIP Latents. arXiv preprint arXiv:2204.06125. https://arxiv.org/abs/2204.06125
- Goodfellow, I., et al. (2014). Generative Adversarial Networks. arXiv preprint arXiv:1406.2661. https://arxiv.org/abs/1406.2661
- OpenAI. (2023). GPT-4 Technical Report. https://arxiv.org/abs/2303.08774